Introduction to Data Analysis with R
Live Online: groups/1-on-1 sessions
Description
At the end of the course, the participant is expected to have acquired the statistical fundamentals to:
-
Visualize and describe data.
-
Calculate and interpret descriptive measures.
-
Calculate and interpret probabilities of events.
-
Recognize and apply the appropriate estimation methods to infer about the population.
-
Recognize and apply appropriate hypothesis tests to infer about the population.
-
Use computational tools in R for data analysis.
This course provides an introduction to Basic Statistics for those with little or no experience in statistics. Upon completion of this course, the participant will be able to apply and understand statistical concepts, apply data visualization, and inferential methods in data analysis.
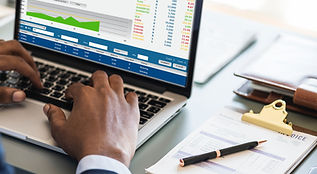
Level: introductory
Delivery: Live Online
Weeks: 4
Prerequisites: none
Certification: yes
Learning Objectives
Instructor
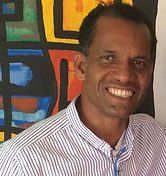
Henry Mendoza Rivera
Henry Mendoza Rivera is the founder and president of mystatistician.com. He is the author of several online statistics courses. He was a professor in the Department of Statistics at The University of Wisconsin-Madison, Edgewood College, San Diego Community College District, and The National University of Colombia-Bogotá. He was the former director of the National Directorate of the Academic Innovation-National University of Colombia. Currently working as a professor for the MSc in Data Science from the National University-San Diego (USA). He has provided statistical consultation and training to INVIMA - Colombia National Food and Drug Surveillance Institute, Liberty Insurance-Colombia, Colombian Institute of Family Welfare (ICBF). He has been a guest in online education in statistics at Masachusset Technology Institute (MIT), as well as at different statistics Workshops, and has presented papers at international conferences.
Course Content
Week 1
Data Visualization and Exploration
-
Categorical variables
-
A quantitative variable: Shape and trend measures.
-
A quantitative variable: Dispersion measures.
-
Boxplot.
-
Two quantitative variables: Scatter plot and correlation.
-
Two quantitative variables: Linear regression.
Week 3
Hypothesis Tests
-
Hypothesis test for the mean and proportion: a group
-
Hypothesis test for the mean and proportion: two groups.
Week 2
Confidence Intervals
-
Sampling distributions
-
Interpretation of confidence intervals
-
Bootstrap Confidence Intervals
Week 4
Inference for Multiple Parameters
-
Chi-square tests for categorical variables.
-
ANOVA to compare means.